AI can create customer value in a broad range of domains, and can be used to take products to the next level. Product teams are often able to generate a lot of ideas for how they can use AI. But, only a small percentage of these ideas turn out to be viable since there are considerable risks related to new product ideas. This is especially the case with products based on disruptive technologies, such as AI.
The most important aspects to consider when evaluating an AI idea are:
- Value risk: Will customers buy the product?
- Usability risk: Will the user understand how to use the product?
- Technical feasibility risk: Is the product team able to build a product that solves the problem and creates customer value?
- Business viability risk: Can stakeholders such as marketing, sales, and legal support the product?
The key differentiator for high-performing product teams is that they’re able to assess these risks prior to building and shipping the product. This enables them to explore and innovate more rapidly and efficiently than their competitors.
We’ve seen time and again that it’s especially difficult to evaluate the technical feasibility risk of AI products because the performance of the AI algorithms often will make or break the product idea. And this can be hard to evaluate without conducting extensive testing over several months.
What we mean by “AI performance”
AI performance means the quality of the output produced by the AI, spanning predictions, recommendations, and the generation of new content. It quantifies the extent to which the AI’s output aligns with the expected result. For instance, in the context of a text generative model, AI performance evaluates the relevance and grammatical accuracy of the generated text in comparison to a reference text. In the case of sales forecasting, AI performance measures the accuracy of the AI’s predictions regarding future sales quantities in contrast to the actual realised sales.
A new framework for evaluating technical feasibility
So, how can product teams increase their ability to explore and develop AI products efficiently? For starters, they can use the following framework, which consists of two main questions and guidelines on how to answer them:
- How performant does the AI need to be? If low AI performance is sufficient to create customer value, then the risk of technical infeasibility is greatly reduced, and vice versa.
- How performant is the AI likely to be? This can be answered by looking at the characteristics of the problem to be solved and the availability of data.
The answer to these questions will serve as good input to the technical feasibility risk of the product.
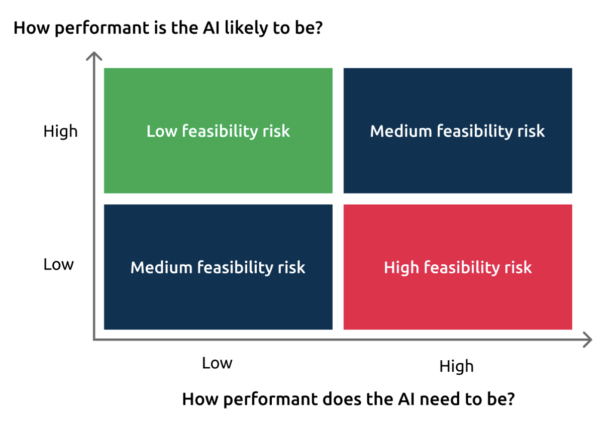
How performant does the AI need to be?
When deciding what makes the AI “good enough” to create customer value, the first thing to consider is the importance of the problem you’re solving. Is it a problem that customers really care about? If the problem is unimportant, it sets an upper limit to how valuable the product can be.
Second, consider how the end users are solving the problem today. This determines the alternative cost of using AI. Customers might be able to solve the problem in a very satisfying way, without the support of AI. Or, the problem might be nearly impossible to solve without some sort of AI-smartness.

Nice-to-have feature: The customer isn’t able to solve the problem to a satisfying degree with today’s solution, so AI could create some value without being very performant. However, the fact that the problem isn’t really important limits the potential of the product.
Bad case for AI: Product ideas in this quadrant have a very high feasibility risk and are unlikely to create value. This is because they’re solving a problem that’s unimportant for the customer, who already has a satisfactory solution.
Risky: As the name suggests, these ideas can potentially be very valuable, but there’s a high degree of uncertainty. Whether to proceed with these ideas or not depends on how performant the AI is likely to be.
Sweet spot: Product ideas in this category are highly likely to create customer value. They solve important problems to which there is currently no feasible solution, making the customer likely to try anything that works. As a result, the success of the product idea depends less on how performant the AI is likely to be.
Test case: Apple Siri
Imagine you’ve asked Siri to “open Spotify”, and Siri responds by opening the Notify app. Many iPhone users have experienced something similar. This example illustrates that a product can be both very performant and create zero value at the same time.
If we look at the prediction in isolation, it’s actually very accurate. Out of the endless number of alternative commands you could give, it interprets it almost perfectly. Unfortunately, the value of Siri guessing a command almost correctly is zero because the current solution approach is very feasible: you can open Spotify in just a few clicks. So, that puts Siri in the “Bad case for AI” category, since it’s solving a relatively unimportant problem that’s already solved to a satisfying degree by an alternative approach (for most users, at least).
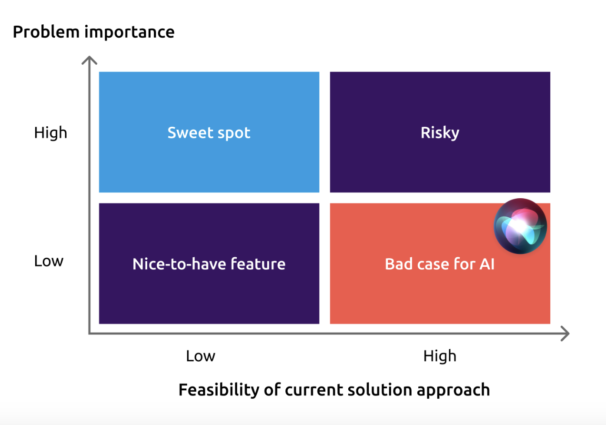
How performant is the AI likely to be?
Now that you understand how performant the product needs to be in order to create value, it’s time to evaluate the most likely performance level of the product. In general, it’s very difficult to predict the performance of an AI algorithm without actually building and testing it. This is because these algorithms depend on the availability of correct data, and it’s hard to say how much data is enough. It also depends on the type of AI algorithm we apply – ranging from supervised machine learning models to mathematical optimisation heuristics.
It is possible, however, to say something about the likeliness of a high or low level of performance based on the two following factors:
- The degree of problem isolation
- The degree of problem standardisation (scalability)
The degree of problem isolation
The degree of isolation impacts the number of factors contributing to the problem that we want the AI to solve. If the problem is isolated, there are fewer contributing factors, which makes it more feasible to model and solve. On the other hand, if the problem is impacted by a large number of factors, then it will be almost impossible for the AI to take all of them into account, making the solution quality worse.
Let’s consider an AI product that predicts future oil prices. There are millions of variables that impact the price of oil, including war, financial crises, and so on. The AI will have access to a very small portion of the factors that impact the price, which limits the prediction accuracy. This doesn’t mean that making a product that predicts oil prices can’t create value, since limited accuracy is often better than nothing. But, keep in mind that there will be limits to how well it will perform.
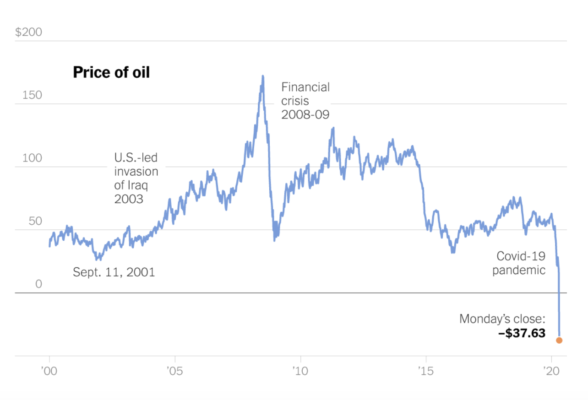
Source: New York Times
Now let’s consider an AI product that converts paper invoices into e-invoices. Because scanning of invoices is determined solely by the content of the invoice, you’d have access to all the factors that decide the correct way to do the conversion. There are no external factors that affect how the model should interpret the content of the paper invoice. So, you can expect relatively high accuracy.

Source: Fyle
The degree of problem standardisation
In the early phases of product discovery, product teams typically build simple AI prototypes and test them on data from a few customers. In this phase, it’s extremely important to proceed with scalability in mind. Often during testing of the AI-based prototypes, teams will want to add additional data sources in order to improve the solution quality. And the data sources required vary from customer to customer. This means they’ll potentially need to create custom machine learning data pipelines and model pipelines for each customer, which makes the product tailored to specific customers. This again limits scalability. So, be critical of product ideas where the product success relies on using customer-specific data sources.
Final thoughts
All in all, evaluating technical feasibility isn’t black and white. It’s impossible to say for certain whether a product idea will end up successful or not without actually building and testing the product in the market. But, having a basis to consider technical feasibility increases the likelihood that product teams make the correct decisions.